With the latest radio telescopes recording more than 200 terabytes of data daily, our astronomers have to find a way to sift through data quickly and effectively. That's why they've created computers with brains.
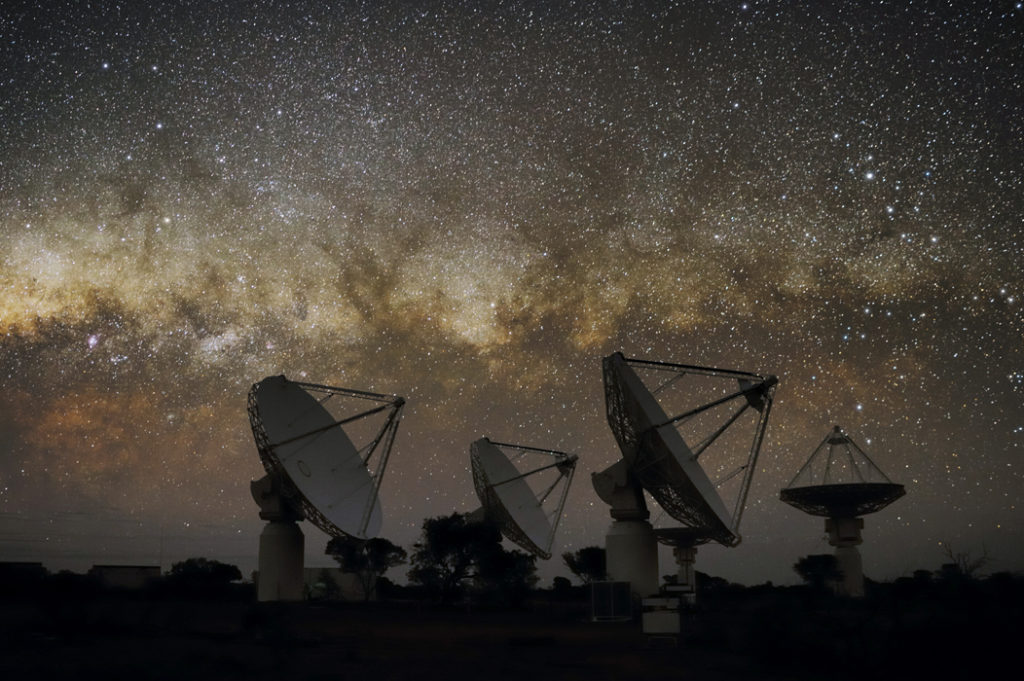
CSIRO's new telescope, the Australian SKA Pathfinder (ASKAP), standing tall in Western Australia.
Don’t you hate it when you get so much data from your super-powerful telescopes that you have to build a fleet of robot brains to interpret it?!
Credit: Alex Cherney/terrastro.com
Most major astronomical discoveries usually come from what we can call ‘unknown unknowns’ – things that we don’t even know are out there until we accidentally stumble across them.
These discoveries are often the result of someone observing the universe in a way it hasn’t been observed before. With several next-generation telescopes being built, including the international Square Kilometre Array (SKA), we can see more of the universe than ever before.
So there’s an excellent chance that the next major astronomical discovery is being made right now, but with the latest radio telescopes recording more than 200 terabytes of data each day, our astronomers need to develop ways to cut through the data deluge and be able to pick out the important bits.
Brains in space
To solve this problem, Professor Ray Norris from our Astronomy and Space Science team has developed Widefield ouTlier Finder (WTF). WTF is a cloud-based computing system that uses machine learning techniques to ‘teach’ the system all the things that we know about astronomy.
Building an artificial ‘neural network’ involves modelling a computing system on our very own neural network (the brain!). Artificial neurons are built as a mathematical model of their biological namesakes, connected together to mimic how they are connected biologically.
“Once we’ve taught it everything we know, then the trick is to ask it to find the things that we don’t know about, and hopefully find any hidden gems that might be right under our nose.” Ray said.
Computers can learn from their mistakes too
At first, WTF will probably turn up things we forgot to tell it, and it will also find radio interference and instrumental artefacts. As we gradually teach it what these are it will start to recognise truly new objects and phenomena and, more significantly, because of its multidimensional complexity, it will start to learn new things from the data that would have otherwise been invisible to us.
However, the huge advantage of building this type of system is that it provides a machine with a level of intelligence to be able to make connections that traditional programs couldn’t. It can ‘learn’ from its mistakes and achieve more accurate results over time.
A South African ‘Summer Down Under’
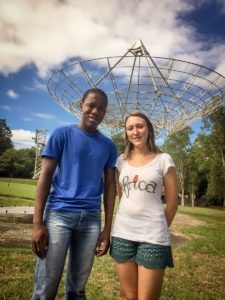
South African students Tokiso Motoai and Katherine James
Ray recently mentored South African student Katherine James as part of our summer vacation scholarship program. Normally open only to Australian students, Katherine and fellow South African student Tokiso Motoai were the inaugural recipients of an initiative jointly funded by SKA South Africa and ourselves.
The program provided opportunities for South African students to travel to Australia and work with our scientists on projects supporting the SKA, our own ASKAP telescope and MeerKAT, the South African SKA precursor telescope.
During his time in Australia, Tokiso worked with Dr George Hobbs to look for synergies between the Parkes radio telescope and MeerKAT for observing pulsars (rapidly spinning neutron stars).
While Katherine was here, she worked with Ray and his team to develop machine learning techniques for analysing the data being captured by ASKAP.
Giving computers the gift of sight
With ASKAP expected to detect about 70 million radio sources courtesy of the Evolutionary Map of the Universe (EMU) survey, the traditional method of cross-matching infrared and radio images by eye is no longer realistic.
To overcome this hurdle, Katherine built a convolutional neural network that’s able to automate the classification and cross-identification process by mimicking the organisation of a human’s visual cortex, which we use to process images. The artificial network ‘sees’ the same images a human would, and is able to learn and process the images in a much faster and more automated manner than a trained human.
“Katherine’s project on applying machine learning techniques to radio astronomy challenges is very important both for ASKAP and for the South African MeerKAT telescope. I know my South African colleagues are exploring similar issues, so I hope that this vacation scholarship program might trigger productive long-term collaborations between CSIRO and South African astronomers,” Ray said.
Find out more about how we’re contributing to the international SKA project.
3rd April 2017 at 3:26 pm
Very exciting, and a little bit scary. A big learning computer won’t miss a beat and will get better the more it learns, exponentially.